Combining PyTorch RNN, TCNN, and Deep Neural Network Models for Production

In recent years, deep neural networks (DNNs) have become increasingly popular for a wide range of tasks, from image classification and object detection to natural language processing and speech recognition. However, deploying DNNs in production can be a challenging task, especially for large-scale models that require significant computational resources.
One way to address this challenge is to use a combination of different types of neural networks, such as recurrent neural networks (RNNs) and temporal convolutional neural networks (TCNNs). RNNs are particularly well-suited for processing sequential data, while TCNNs are effective for capturing temporal dependencies in data. By combining these two types of networks, it is possible to create models that are both powerful and efficient.
In this article, we will provide a comprehensive guide to combining PyTorch RNN, TCNN, and DNN models for production. We will cover the benefits of using these models, the challenges involved, and the best practices for deploying them in production environments.
4.4 out of 5
Language | : | English |
File size | : | 4877 KB |
Text-to-Speech | : | Enabled |
Screen Reader | : | Supported |
Enhanced typesetting | : | Enabled |
Print length | : | 421 pages |
There are several benefits to using a combination of PyTorch RNN, TCNN, and DNN models for production. These benefits include:
- Improved performance: By combining different types of neural networks, it is possible to create models that are more accurate and efficient than models that use a single type of network.
- Reduced computational cost: By using a combination of different types of networks, it is possible to reduce the computational cost of training and deploying models.
- Increased flexibility: By using a combination of different types of networks, it is possible to create models that can be adapted to a wider range of tasks.
There are also several challenges involved in combining PyTorch RNN, TCNN, and DNN models for production. These challenges include:
- Model complexity: Combining different types of neural networks can lead to models that are more complex and difficult to train.
- Data requirements: Training models that combine different types of neural networks requires a large amount of data.
- Deployment complexity: Deploying models that combine different types of neural networks can be complex and time-consuming.
There are several best practices for combining PyTorch RNN, TCNN, and DNN models for production. These best practices include:
- Start with a simple model: When combining different types of neural networks, it is important to start with a simple model. This will help you to understand the basic principles of how these networks work together.
- Use a pre-trained model: If possible, use a pre-trained model as the starting point for your own model. This will save you time and effort, and it will also help you to achieve better results.
- Tune the model parameters: Once you have a basic model, you can tune the model parameters to improve its performance. This can be done by using a variety of techniques, such as grid search and random search.
- Deploy the model using a cloud service: Once you have a trained model, you can deploy it using a cloud service. This will allow you to scale your model to meet the demands of your production environment.
Combining PyTorch RNN, TCNN, and DNN models can be a powerful way to improve the performance, efficiency, and flexibility of your deep learning models. However, there are also several challenges involved in combining these types of models. By following the best practices outlined in this article, you can overcome these challenges and successfully deploy your models in production.
4.4 out of 5
Language | : | English |
File size | : | 4877 KB |
Text-to-Speech | : | Enabled |
Screen Reader | : | Supported |
Enhanced typesetting | : | Enabled |
Print length | : | 421 pages |
Do you want to contribute by writing guest posts on this blog?
Please contact us and send us a resume of previous articles that you have written.
Fiction
Non Fiction
Romance
Mystery
Thriller
SciFi
Fantasy
Horror
Biography
Selfhelp
Business
History
Classics
Poetry
Childrens
Young Adult
Educational
Cooking
Travel
Lifestyle
Spirituality
Health
Fitness
Technology
Science
Arts
Crafts
DIY
Gardening
Petcare
Elizabeth Dupart
Denise May Levenick
Douglas P Fry
Law School Admission Council
Elizabeth May
Warwick Deeping
Kathy Woods
George Daniel
Darcy Lever
Kaplan Test Prep
Dr Monika Chopra
Leslie A Sams
Leah Hazard
Mark Young
Luc Mehl
Lee Jackson
Kevin A Morrison
Narain Moorjani
Lina K Lapina
Albert Rutherford
Marco Wenisch
Wyatt Mcspadden
Della Ata Khoury
Ron Senyor
Jessica Howard
Michael Blastland
Lauren Manoy
Max Lucado
Norman Thelwell
Graham Hancock
Tom Bass
Earl G Williams
Francis Glebas
Tey Meadow
Trevor Thomas
Terry Laughlin
Michael Chatfield
Cassandra Mack
Katherine D Kinzler
Neville Goddard
Mo Gawdat
Pam Flowers
Philip Purser Hallard
Winky Lewis
Jeffrey L Kohanek
John H Cunningham
Lottie Bildirici
Peter Bodo
Lew Freedman
Paige Powers
Jeff Scheetz
Jason Runkel Sperling
Dorothy Canfield Fisher
Elena Paige
Orangepen Publications
Henry Malone
Kerry H Cheever
David Wilber
Karen J Rooney
Huberta Wiertsema
John Jamieson
Matthew Warner Osborn
Mary Pagones
Christine Mari Inzer
Jasmine Shao
Sylvia Williams Dabney
Bruce Watt
Donna R Causey
Joanne V Hickey
Sammy Franco
Peterson S
Oliver Sacks
Clement Salvadori
Emily Writes
Robert Moor
Katharine Mcgee
Lsat Unplugged
Doug Cook
Byron L Reeder
Philippe Karl
Jim Warnock
Jared Diamond
Frederick Jackson Turner
Richard Barrett
Ken Schwaber
Dr Tommy John
Jennifer Rose
Db King
Howard E Mccurdy
Aaron Reed
Charles Salzberg
Shaunti Feldhahn
Susan Garcia
Gregory A Kompes
Marc Bona
J R Harris
Scott Cawthon
Lynette Noni
Charles Staley
Sallyann Beresford
Jesse Romero
Richard W Voelz
Maria Van Noord
Paul Lobo
Ransom Riggs
Nick Gamis
Craig Callender
Eric P Lane
Ashley Eckstein
Cameron Mcwhirter
Maha Alkurdi
Buddy Levy
Carmen Davenport
Bruce Sutherland
Robert Edward Grant
Julietta Suzuki
Ronald T Potter Efron
Kacen Callender
Helen Webster
Joanne Kimes
Pia Nilsson
Konstantinos Mylonas
Sarah Ockwell Smith
Jack Canfield
Freya Pickard
Jim Kempton
Leslie R Schover
Veronica Eden
John Moren
Dory Willer
Jennifer L Scott
Ken Sande
Thomas Deetjen
Guy Grieve
Jim West
Meg Cabot
Wayne Coffey
Carrie Hope Fletcher
Dianne Maroney
Matt Mullenix
Lisa Hopp
John R Mabry
Skip Lockwood
Stacey Rourke
Jess J James
Chris Bonington
Mark Hansen
Helen Zuman
Richard Drake
Robert D Gibbons
Lisa Feldman Barrett
Wilhelm Reich
John Burroughs
Kevin Houston
Valerie Poore
Julie Golob
Duncan Steel
Julia Ann Clayton
S M Kingdom
John H Falk
Fiona Beddall
Thomas Achatz
Ralph Galeano
Max Lugavere
Bryce Carlson
Nick Littlehales
Stephen Harrison
Simon Michael Prior
William L Sullivan
Susan Orlean
Martina Mcbride
Stella Cottrell
Janet Evans
Kevin Panetta
Caitlyn Dare
Roger J Davies
Ian Tuhovsky
Fmg Publications Special Edition
Steve Barrett
Paul A Offit
Simon A Rego
Nicholas Tomalin
Lily Field
Mark Stavish
Rita Golden Gelman
C S Lewis
David Tanis
Malika Grayson
Nikki Carroll
Marcia Scheiner
Elmer Keith
Healthfit Publishing
Emma Warren
Jamie Marich
Robert Larrison
Diane Lindsey Reeves
Stephen King
Sherri L Jackson
Zachery Knowles
Nedu
E W Barton Wright
R Scott Thornton
Chris Morton
Joseph Correa
Robert Garland
Shea Ernshaw
Troy Horne
Maxine A Goldman
Erin Macy
Meriwether Lewis
Lee Alan Dugatkin
Heather Balogh Rochfort
Creek Stewart
Michael A Tompkins
Craig Martelle
Gary Lewis
Suzanne Young
John Vince
Bruce W Harris
Cecil B Hartley
Joel Best
Meghan Daum
Max Prasac
Vivian Foster
Matthew Marchon
Tim Glover
W D Wetherell
William Rathje
Destiny S Harris
Sarah Prager
Tim Freke
Paul Murdin
Lucy Cooke
Sheri Morehouse
John Kretschmer
Heather Jacobson
Tamara Ferguson
Sue Elvis
Dian Olson Belanger
Maurice J Thompson
Shelby Hailstone Law
Elaine Tyler May
Michael Mewshaw
Fern Schumer Chapman
Tibor Rutar
Larry Larsen
Eric R Dodge
Bruce Van Brunt
Ashley Christensen
Tony E Adams
Kelly Rowland
Sara Gaviria
Kyra Phillips
Lianna Marie
Judith Merkle Riley
Mathew Orton
David Martin
Rand Cardwell
Elizabeth Anne Wood
Mary C Townsend
Shawna Richer
Valliappa Lakshmanan
Michael Lear Hynson
Ivan Gridin
Jill Angie
Ivy Hope
Scarlett V Clark
Hecateus Apuliensis
Martin Davies
Natasha Daniels
Cornelia Pelzer Elwood
Sterling Test Prep
Anthony Camera
Brandy Colbert
Emily Lowry
Upton Sinclair
Lewis Kirkham
Laurie Notaro
Jim Wiese
Ed Housewright
Jane Brocket
Jennifer Appel
Rob Pate
William Ian Miller
Lily Raff Mccaulou
Joyceen S Boyle
Marisa Peer
William M Baum
Michelle Travis
Sarah Baker
Erin Beaty
Stian Christophersen
Collins Easy Learning
Jacob Erez
Oprah Winfrey
Tina Schindler
C J Archer
Tyler Trent
Tanya Hackney
Steven Kerry Brown
Keith Brewer
Issai Chozanshi
James Goi Jr
Rowena Bennett
Donna Goldberg
Jonathan T Gilliam
Dvora Meyers
Peter J D Adamo
Sarah Berman
Nawuth Keat
David Eagleman
Mona Bijjani
Natasha Ngan
Nigel Cawthorne
Jessica Holsman
L W Jacobs
Caroline Manta
Kevin Howell
C M Carney
John M Marzluff
Kendall Rose
Frank Muir
James Beard
Graham Norton
Don Allen Jr
Mcgraw Hill
David Cannon
Sheila A Sorrentino
Paul Rabinow
Ned Feehally
Marc Van Den Bergh
Melissa A Priblo Chapman
William Wood
Justin Coulson
Richard C Francis
Deirdre V Lovecky
Sam Priestley
Winslow Tudor
John Flanagan
Jimmy Chin
Ian Leslie
Elisabeth Elliot
Tim S Grover
James W Anderson
Barbara Acello
Nicola Yoon
Gary Mayes
Maren Stoffels
Richard Harding Davis
Ron Rapoport
Chris Pountney
Niels H Lauersen
Declan Lyons
Scott Mactavish
Linda Rosenkrantz
Eugenia G Kelman
Martina D Antiochia
Vladimir Lossky
Jim Al Khalili
Jeff Belanger
John J Ratey
Carole Bouchard
Michael Gurian
Nicolas Bergeron
Chad Eastham
Sophie D Coe
Ronit Irshai
Michael D Alessio
Celeste Headlee
Kat Davis
Jack Disbrow Gunther
Rebecca Musser
Hibiki Yamazaki
R L Medina
Steve Guest
James Miller
Shyima Hall
Dan Romanchik Kb6nu
Don S Lemons
Carlos I Calle
Hugh Aldersey Williams
John Grehan
Dave Rearick
Sarah Jacoby
Richard Henry Dana
Pamela Weintraub
Elizabeth Laing Thompson
Lingo Mastery
Bruce Maxwell
Carrie Marie Bratley
Veronica Roth
Ira K Wolf
Lois Lowry
William Ellet
Edward Humes
E Ink Utilizer
Vincent Chidindu Asogwa
Leah Zani
Ivar Dedekam
Marla Taviano
Kathleen Flinn
Lynn Butler Kisber
Jen Howver
Thomas Carothers
Robert A Cutietta
Sophie Messager
Shmuel Goldberg
Phil Williams
J R Rain
Albert Jeremiah Beveridge
Cal Newport
Charu C Aggarwal
Joe Baker
Scott Hartshorn
Dave Bosanko
Rick Trickett
David Nathan Fuller
Phil Bourque
Om Krishna Uprety
Matt Racine
Melanie Anne Phillips
Romola Anderson
Steven W Dulan
C F Crist
Richard Bullivant
Dan Flores
Jim Supica
M E Brines
Trevelyan
Bryan Litz
Greg W Prince
J D Williams
Silvia Dunn
Matt Baglio
Olivier Doleuze
Kindle Edition
Robert Walker
Tyler Burt
Susan Frederick Gray
Scott Mcmillion
Leon Speroff
Theodora Papatheodorou
Melissa Mullamphy
Dick Hannula
Daniel Prince
Mark Stanton
Tom Cunliffe
J Maarten Troost
Victoria Honeybourne
Dounya Awada
Cathy Glass
Katie Fallon
Ruthellen Josselson
Bunmi Laditan
Michael Tan
Samantha De Senna Fernandes
Tricia Levenseller
Pedro Urvi
Arrl Inc
Kruti Joshi
Caleb J Tzilkowski
David Savedge
The Atavist
Thomas Daniels
Sonia Shah
Megan Miller
Joseph Moss
Dennis Adler
Carol Inskipp
Emt Basic Exam Prep Team
Douglas Preston
Sandra Niche
Martha Finley
Nicola S Dorrington
Mike X Cohen
Matt Price
Pat Rigsby
Charles Sanger
Stedman Graham
Roger Marshall
Dina Nayeri
Nick Tumminello
K C Cole
Light bulbAdvertise smarter! Our strategic ad space ensures maximum exposure. Reserve your spot today!
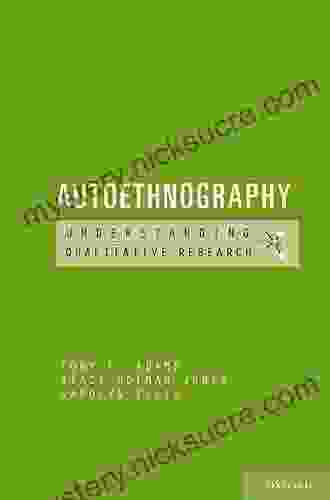

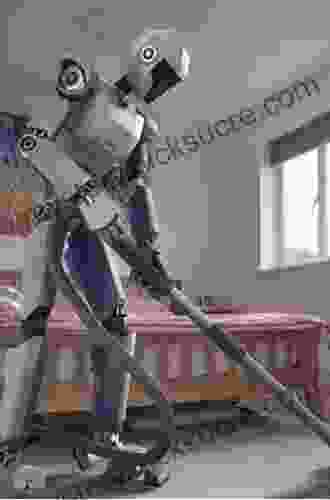

- Jason HayesFollow ·18.6k
- Patrick RothfussFollow ·12.6k
- Gage HayesFollow ·8.1k
- Angelo WardFollow ·7k
- Colton CarterFollow ·14.2k
- Mikhail BulgakovFollow ·9.5k
- Hudson HayesFollow ·9.1k
- Frank ButlerFollow ·18.4k
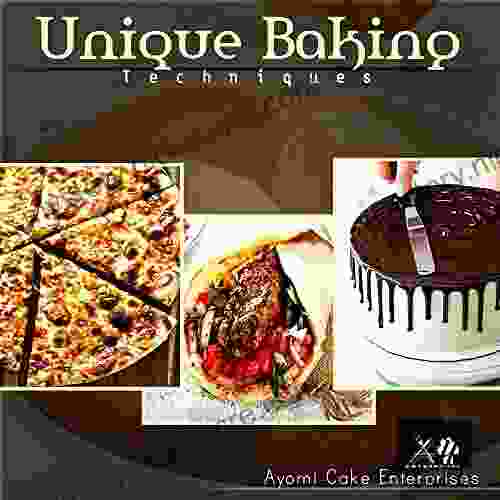

How To Bake In Unique Way: Unleash Your Culinary...
Baking is an art form that transcends the...
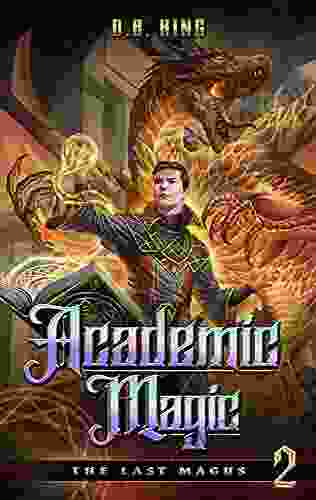

Academic Magic: Unveil the Secrets of The Last Magus
Delve into a Realm of...
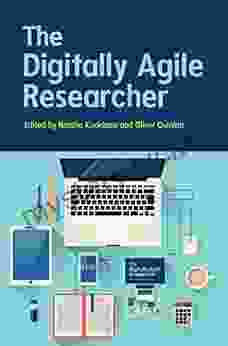

The Digitally Agile Researcher in UK Higher Education:...
In the rapidly...
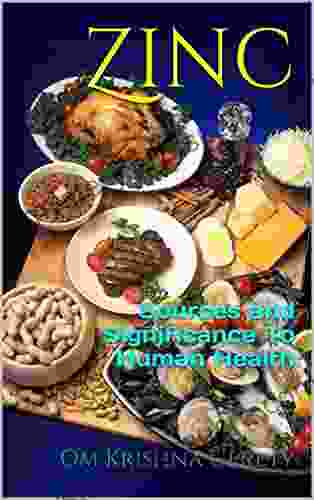

Zinc: Sources And Significance To Human Health
Zinc, an essential trace mineral, plays a...
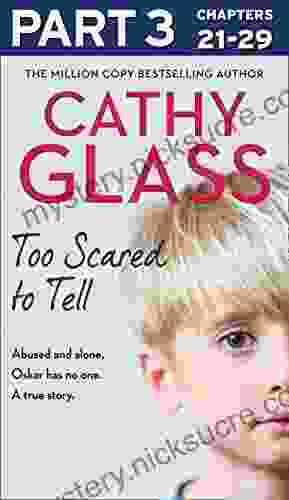

Too Scared to Tell: A Harrowing and Thought-Provoking...
In the realm...
4.4 out of 5
Language | : | English |
File size | : | 4877 KB |
Text-to-Speech | : | Enabled |
Screen Reader | : | Supported |
Enhanced typesetting | : | Enabled |
Print length | : | 421 pages |