Solutions To Common Challenges In Data Preparation Model Building And MLOps

Data preparation, model building, and MLOps are essential steps in the machine learning workflow. However, these processes often come with their own set of challenges that can hinder the success of your machine learning projects. In this article, we will explore the common challenges faced in each of these stages and provide practical solutions to overcome them.
Data Collection and Integration
Collecting and integrating data from multiple sources can be a daunting task. The data may be in different formats, have missing values, or contain errors.
Solutions:
4.6 out of 5
Language | : | English |
File size | : | 22463 KB |
Text-to-Speech | : | Enabled |
Screen Reader | : | Supported |
Enhanced typesetting | : | Enabled |
Print length | : | 410 pages |
- Use data integration tools to automate the process of data collection and integration.
- Define clear data standards and ensure that all data sources adhere to these standards.
- Implement data quality checks to identify and correct errors or missing values.
Data Cleaning and Transformation
Once the data is collected, it needs to be cleaned and transformed to make it suitable for model building. This can involve removing outliers, normalizing data, and creating new features.
Solutions:
- Use data cleaning libraries to automate the process of data cleaning and transformation.
- Apply feature engineering techniques to create new features that are more relevant to the model.
- Visualize the data to identify outliers and other data quality issues.
Data Exploration and Understanding
Before building a model, it is important to explore and understand the data. This can help you identify patterns, relationships, and insights that can inform your model design.
Solutions:
- Use data visualization tools to explore the data and gain insights.
- Perform statistical analysis to identify trends and correlations.
- Collaborate with domain experts to gain a deeper understanding of the data.
Feature Selection and Engineering
Selecting the right features for your model is critical for its performance. Irrelevant or redundant features can hurt the model's accuracy.
Solutions:
- Use feature selection techniques to identify the most relevant and predictive features.
- Apply feature engineering techniques to create new features that are more informative for the model.
- Leverage domain knowledge to select features that are known to be important for the problem you are trying to solve.
Model Training and Tuning
Training and tuning a machine learning model can be a time-consuming and iterative process. It requires careful selection of hyperparameters and strategies to prevent overfitting or underfitting.
Solutions:
- Use automated machine learning tools to streamline the process of model training and tuning.
- Experiment with different hyperparameter settings and evaluate the model's performance on validation data.
- Apply regularization techniques to prevent overfitting and improve the model's generalization ability.
Model Evaluation and Selection
Once a model is trained, it needs to be evaluated to assess its performance. This involves using metrics that are relevant to the problem you are trying to solve.
Solutions:
- Use multiple evaluation metrics to get a comprehensive view of the model's performance.
- Compare the model's performance to that of other models or baselines.
- Consider the business impact of the model's predictions and select the model that best aligns with your goals.
Model Deployment and Monitoring
Deploying a machine learning model into production and monitoring its performance is crucial for ensuring its continuous success.
Solutions:
- Use model deployment tools to automate the process of model deployment.
- Implement monitoring systems to track the model's performance and identify any issues.
- Re-train and update the model as needed to maintain its performance over time.
Data Lineage and Reproducibility
Tracking the lineage of your data and models is essential for understanding how the model was built and for reproducing the results.
Solutions:
- Use data lineage tools to track the origin and transformation of your data.
- Implement version control for your models and data to ensure reproducibility.
- Document the model building process and share it with stakeholders.
Collaboration and Communication
Successful MLOps requires collaboration between data scientists, engineers, and business stakeholders.
Solutions:
- Establish clear communication channels between different teams.
- Use tools for collaboration and knowledge sharing.
- Define roles and responsibilities for each stakeholder involved in the MLOps process.
Data preparation, model building, and MLOps are critical aspects of the machine learning workflow. By addressing the common challenges associated with each of these stages and implementing the solutions outlined in this article, you can streamline your machine learning workflow, improve the performance of your models, and ensure their successful deployment and operation in production.
4.6 out of 5
Language | : | English |
File size | : | 22463 KB |
Text-to-Speech | : | Enabled |
Screen Reader | : | Supported |
Enhanced typesetting | : | Enabled |
Print length | : | 410 pages |
Do you want to contribute by writing guest posts on this blog?
Please contact us and send us a resume of previous articles that you have written.
Fiction
Non Fiction
Romance
Mystery
Thriller
SciFi
Fantasy
Horror
Biography
Selfhelp
Business
History
Classics
Poetry
Childrens
Young Adult
Educational
Cooking
Travel
Lifestyle
Spirituality
Health
Fitness
Technology
Science
Arts
Crafts
DIY
Gardening
Petcare
Mark Stavish
Elmer Keith
Nick Littlehales
Samantha De Senna Fernandes
Thomas Deetjen
Caitlyn Dare
Ian Leslie
Robert A Cutietta
Nicolas Bergeron
Shea Ernshaw
Julie Golob
Heather Balogh Rochfort
Matt Price
Tyler Burt
Charu C Aggarwal
Max Lugavere
Lily Field
Mark Young
Caroline Manta
Jason Runkel Sperling
Douglas Preston
Issai Chozanshi
Neville Goddard
Valerie Poore
Duncan Steel
Wyatt Mcspadden
Hibiki Yamazaki
John H Falk
Jesse Romero
Peter Bodo
Steven Kerry Brown
Lew Freedman
Bruce Maxwell
Nick Gamis
Db King
Kelly Rowland
Richard Henry Dana
Jennifer Appel
David Eagleman
W D Wetherell
Melanie Anne Phillips
Michael Tan
Luc Mehl
Matt Mullenix
Megan Miller
Paul Rabinow
Mark Stanton
Mary Pagones
Winky Lewis
Tanya Hackney
Maria Van Noord
Nawuth Keat
David Wilber
Dian Olson Belanger
Fiona Beddall
Eric P Lane
Craig Martelle
Matthew Marchon
Matt Racine
James Goi Jr
Michael Mewshaw
Barbara Acello
Jeff Scheetz
Elizabeth Anne Wood
Nigel Cawthorne
Leah Hazard
Sheila A Sorrentino
M E Brines
Gregory A Kompes
Creek Stewart
Stephen Harrison
Anthony Camera
Leon Speroff
Daniel Prince
Romola Anderson
Susan Garcia
J R Rain
Craig Callender
Max Lucado
Mona Bijjani
Collins Easy Learning
George Daniel
Graham Hancock
Ron Senyor
C J Archer
Dennis Adler
Sandra Niche
James W Anderson
Cornelia Pelzer Elwood
Janet Evans
Jill Angie
Joyceen S Boyle
Sterling Test Prep
John Moren
Mary C Townsend
Marc Van Den Bergh
Emily Writes
Brandy Colbert
Celeste Headlee
Elisabeth Elliot
Lingo Mastery
John Flanagan
Trevor Thomas
Chris Morton
The Atavist
Matt Baglio
Bruce Van Brunt
Ruthellen Josselson
Zachery Knowles
Lewis Kirkham
Robert Moor
Ed Housewright
L W Jacobs
S M Kingdom
Elizabeth May
Jack Canfield
Kendall Rose
Laurie Notaro
Bruce Sutherland
Donna Goldberg
Dorothy Canfield Fisher
Jeffrey L Kohanek
Natasha Ngan
Michael Chatfield
Della Ata Khoury
Meg Cabot
Eugenia G Kelman
Scarlett V Clark
John H Cunningham
Robert D Gibbons
Jacob Erez
Jim West
Vincent Chidindu Asogwa
K C Cole
Scott Cawthon
Dan Romanchik Kb6nu
Roger Marshall
Kat Davis
Ned Feehally
Nick Tumminello
Emt Basic Exam Prep Team
Doug Cook
Michael D Alessio
Sara Gaviria
Scott Mcmillion
Narain Moorjani
Shmuel Goldberg
Cassandra Mack
Kaplan Test Prep
Pam Flowers
Stedman Graham
Pia Nilsson
Dvora Meyers
Marc Bona
Cecil B Hartley
Justin Coulson
Vladimir Lossky
Jennifer L Scott
Ron Rapoport
Michael Blastland
Rick Trickett
David Savedge
Joel Best
Dina Nayeri
Mark Hansen
Ken Schwaber
Mike X Cohen
Elizabeth Dupart
Dory Willer
Vivian Foster
Shyima Hall
Larry Larsen
Theodora Papatheodorou
James Beard
Maxine A Goldman
Helen Zuman
Katherine D Kinzler
Philip Purser Hallard
Ian Tuhovsky
Linda Rosenkrantz
Lsat Unplugged
Jasmine Shao
Henry Malone
Joanne V Hickey
William L Sullivan
Martina Mcbride
Earl G Williams
John J Ratey
Max Prasac
Carmen Davenport
Eric R Dodge
Shaunti Feldhahn
Lynn Butler Kisber
Arrl Inc
Paul Murdin
C F Crist
Maurice J Thompson
Rebecca Musser
Kyra Phillips
Bruce Watt
Leslie R Schover
Michael A Tompkins
Dick Hannula
E W Barton Wright
Jim Al Khalili
Jonathan T Gilliam
Steven W Dulan
Pat Rigsby
Orangepen Publications
C M Carney
Om Krishna Uprety
Stephen King
Bunmi Laditan
John Grehan
Thomas Achatz
Pamela Weintraub
Marla Taviano
Maren Stoffels
Fern Schumer Chapman
Aaron Reed
Terry Laughlin
Jamie Marich
Sylvia Williams Dabney
Byron L Reeder
Nedu
Wilhelm Reich
Declan Lyons
Shelby Hailstone Law
Jim Supica
Valliappa Lakshmanan
James Miller
Robert Edward Grant
Cameron Mcwhirter
Dan Flores
Susan Frederick Gray
Law School Admission Council
Joseph Moss
Lucy Cooke
Carole Bouchard
Philippe Karl
Francis Glebas
Elizabeth Laing Thompson
Leslie A Sams
Lisa Feldman Barrett
Jennifer Rose
Martin Davies
Deirdre V Lovecky
Don S Lemons
Tony E Adams
Troy Horne
Freya Pickard
Rob Pate
Rand Cardwell
Mathew Orton
Suzanne Young
Cathy Glass
Meghan Daum
Lianna Marie
Nicola Yoon
Kevin Panetta
Jessica Holsman
Silvia Dunn
Oprah Winfrey
Guy Grieve
Dr Tommy John
Lee Alan Dugatkin
Konstantinos Mylonas
Frank Muir
Cal Newport
Carrie Marie Bratley
Kevin Houston
Roger J Davies
Charles Salzberg
Marcia Scheiner
Nicholas Tomalin
Steve Guest
Veronica Roth
Ivan Gridin
R L Medina
Ralph Galeano
Tamara Ferguson
Rita Golden Gelman
Fmg Publications Special Edition
William Ian Miller
Tom Cunliffe
Dianne Maroney
Tibor Rutar
Sophie Messager
Richard Drake
Julietta Suzuki
Richard Bullivant
Lisa Hopp
Darcy Lever
Peterson S
Tim Freke
J Maarten Troost
Phil Bourque
Ronit Irshai
Melissa Mullamphy
Douglas P Fry
Peter J D Adamo
Sam Priestley
Lottie Bildirici
Ashley Eckstein
David Cannon
Olivier Doleuze
Martha Finley
Edward Humes
Chris Bonington
Lauren Manoy
Chris Pountney
Nicola S Dorrington
Winslow Tudor
Bryce Carlson
Sallyann Beresford
Norman Thelwell
Caleb J Tzilkowski
Sarah Berman
Christine Mari Inzer
Hecateus Apuliensis
Rowena Bennett
Simon A Rego
Joe Baker
Judith Merkle Riley
Victoria Honeybourne
Kindle Edition
Meriwether Lewis
Susan Orlean
R Scott Thornton
Tim S Grover
Kruti Joshi
Michael Lear Hynson
William Wood
Nikki Carroll
John Vince
Emily Lowry
Ivar Dedekam
John Jamieson
Kevin A Morrison
Carrie Hope Fletcher
Emma Warren
Steve Barrett
Skip Lockwood
E Ink Utilizer
Ransom Riggs
Lina K Lapina
Dr Monika Chopra
Jim Warnock
Albert Jeremiah Beveridge
Warwick Deeping
Jim Wiese
Trevelyan
Albert Rutherford
Tey Meadow
Frederick Jackson Turner
Gary Lewis
Healthfit Publishing
Wayne Coffey
C S Lewis
Tom Bass
Robert Garland
Lois Lowry
Carol Inskipp
Sarah Ockwell Smith
Katharine Mcgee
Scott Mactavish
Heather Jacobson
Kathleen Flinn
J D Williams
Ken Sande
Marisa Peer
Greg W Prince
Charles Sanger
Richard C Francis
Martina D Antiochia
Shawna Richer
Elaine Tyler May
Keith Brewer
Tricia Levenseller
Sherri L Jackson
Jared Diamond
Ira K Wolf
Dave Bosanko
Michael Gurian
Elena Paige
Julia Ann Clayton
Donna R Causey
William Rathje
Robert Larrison
John Kretschmer
Lily Raff Mccaulou
Paul Lobo
Stella Cottrell
David Nathan Fuller
Helen Webster
Clement Salvadori
Erin Beaty
Jeff Belanger
Carlos I Calle
Sonia Shah
Sue Elvis
Gary Mayes
Sarah Jacoby
William M Baum
Melissa A Priblo Chapman
Robert Walker
Marco Wenisch
Paige Powers
Ashley Christensen
Malika Grayson
Dave Rearick
Stian Christophersen
Maha Alkurdi
Charles Staley
Jimmy Chin
Erin Macy
Sophie D Coe
Upton Sinclair
Scott Hartshorn
Karen J Rooney
Chad Eastham
Jack Disbrow Gunther
John R Mabry
Thomas Daniels
David Tanis
Denise May Levenick
Tim Glover
Richard Harding Davis
Katie Fallon
Veronica Eden
Kerry H Cheever
Michelle Travis
Diane Lindsey Reeves
Jessica Howard
Don Allen Jr
Sammy Franco
Natasha Daniels
Sarah Prager
Thomas Carothers
Tina Schindler
Buddy Levy
Jess J James
Sheri Morehouse
Mo Gawdat
Lee Jackson
Jim Kempton
Hugh Aldersey Williams
Lynette Noni
Richard W Voelz
Richard Barrett
John Burroughs
Jen Howver
Kathy Woods
Sarah Baker
Niels H Lauersen
J R Harris
Tyler Trent
Jane Brocket
Leah Zani
William Ellet
Destiny S Harris
David Martin
Kevin Howell
Phil Williams
Simon Michael Prior
Dounya Awada
Kacen Callender
Joseph Correa
Paul A Offit
Stacey Rourke
John M Marzluff
Huberta Wiertsema
Ivy Hope
Bruce W Harris
Howard E Mccurdy
Joanne Kimes
Matthew Warner Osborn
Graham Norton
Pedro Urvi
Bryan Litz
Mcgraw Hill
Ronald T Potter Efron
Oliver Sacks
Light bulbAdvertise smarter! Our strategic ad space ensures maximum exposure. Reserve your spot today!
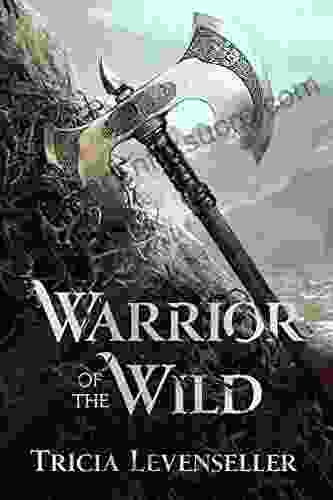

- Ken FollettFollow ·15.9k
- Forrest ReedFollow ·9.2k
- August HayesFollow ·8.1k
- Jean BlairFollow ·10.6k
- Kevin TurnerFollow ·10.6k
- Roger TurnerFollow ·18.7k
- Gus HayesFollow ·17.9k
- Esteban CoxFollow ·12.1k
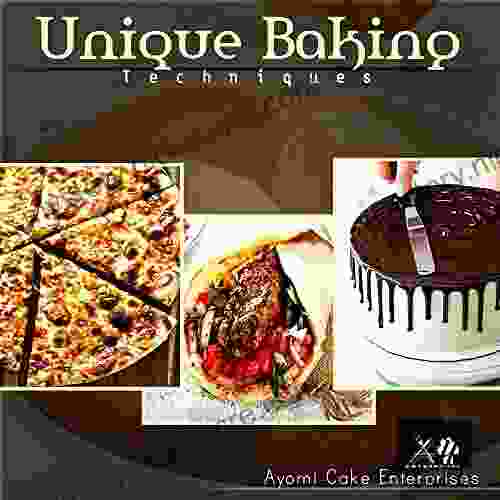

How To Bake In Unique Way: Unleash Your Culinary...
Baking is an art form that transcends the...
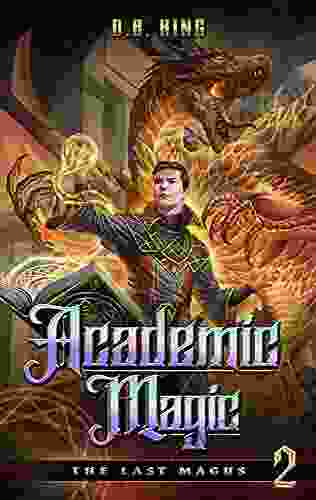

Academic Magic: Unveil the Secrets of The Last Magus
Delve into a Realm of...
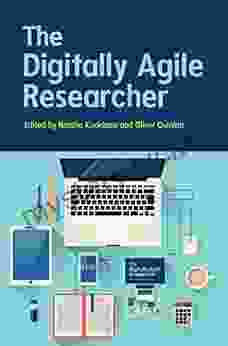

The Digitally Agile Researcher in UK Higher Education:...
In the rapidly...
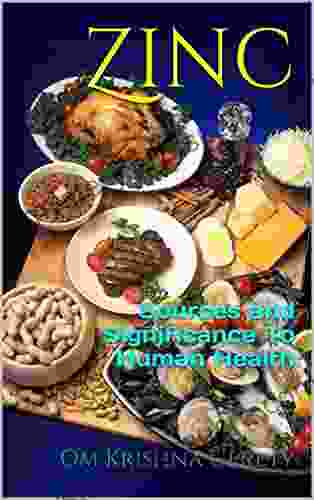

Zinc: Sources And Significance To Human Health
Zinc, an essential trace mineral, plays a...
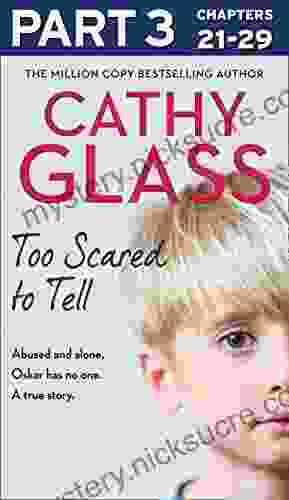

Too Scared to Tell: A Harrowing and Thought-Provoking...
In the realm...
4.6 out of 5
Language | : | English |
File size | : | 22463 KB |
Text-to-Speech | : | Enabled |
Screen Reader | : | Supported |
Enhanced typesetting | : | Enabled |
Print length | : | 410 pages |